Allchemy’s drug-discovery platform combines state-of-the-art computational synthesis with AI algorithms to predict molecular properties. Within minutes, Allchemy creates thousands of synthesizable lead candidates meeting user-defined profiles of drug-likeness, affinity towards specific proteins, toxicity, and a range of other physical-chemical measures. At the push of a button, drug-like scaffolds are created de novo or evolved from user-defined fragments; syntheses are ranked for efficiency and greenness, and are propagated from either user-specified substrates, AI-suggested chemicals, or renewable resources.
Technology
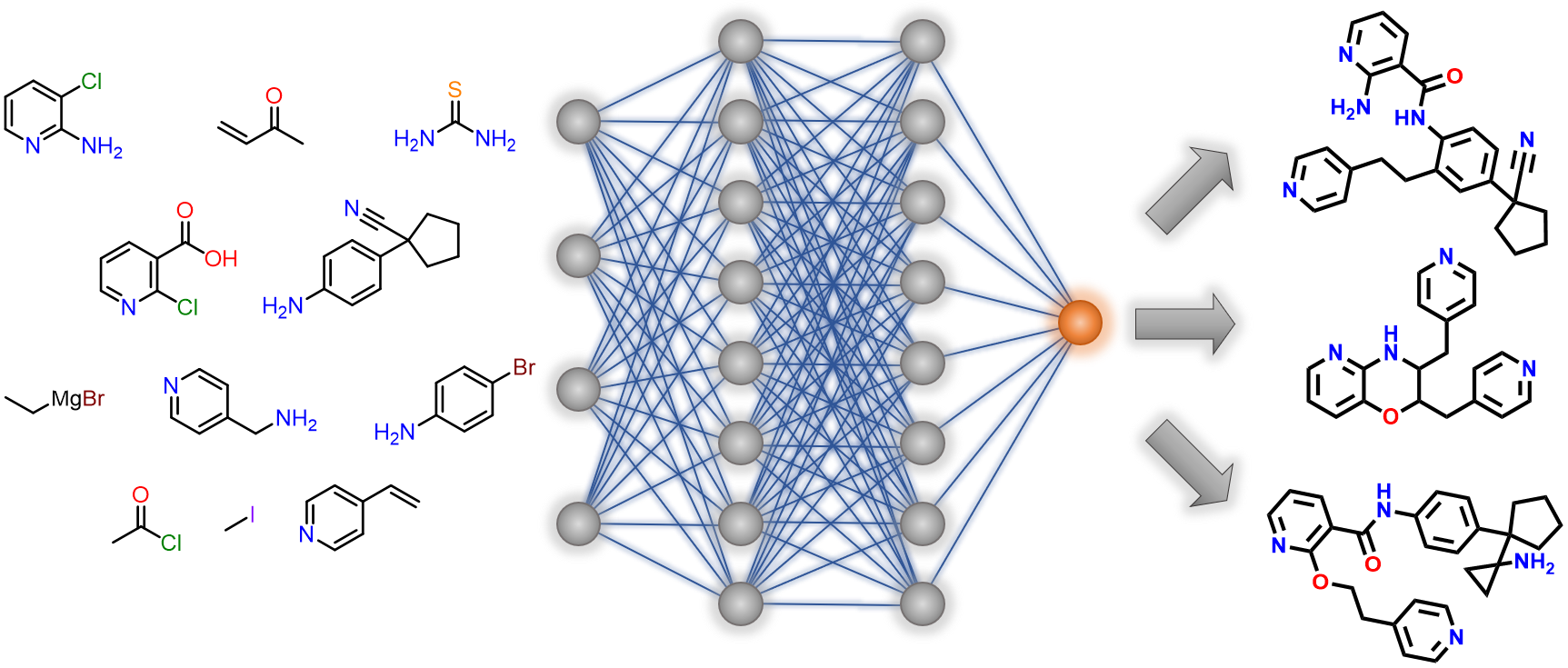
Some of our tools are freely available for you to try. For example, the use of Allchemy’s “Life” module with which to explore prebiotic reactions is available at LINK (short youtube summary is here for detailed user manual see SI to our Science paper). Another freeware illustrates the performance of our AI algorithms to predict molecular properties – at this link, you can play with the tool to predict pKa’s of C-H acids (for details, see JACS). These and other modules are integrated into Allchemy’s professional WebApp – to learn more, please arrange a demo at .
Allchemy encompasses the entire resource-to-drug design process and has been used in academic, corporate and classified environments worldwide to:
People
We are a team of synthetic and medicinal chemists and computer-specialists at the forefront of modern “chemical AI” revolution. Some of us has previously developed the Chematica retrosynthesis platform (cf. recent Nature paper). We have been working closely with both the private sector as well as US Government agencies (especially DARPA).
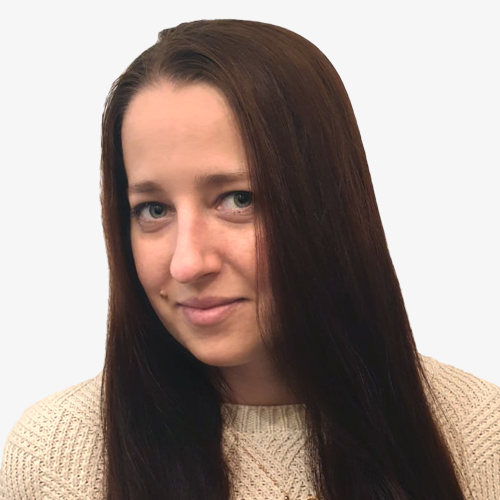
Agnieszka Wołos, PhD
Senior Chemist, in silico drug discovery
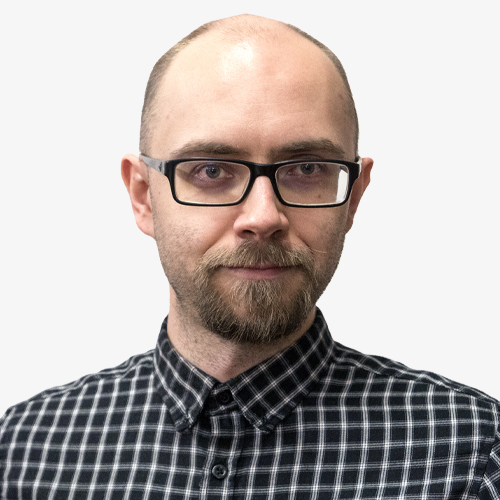
Karol Molga, PhD
Senior Chemist, computational reaction design
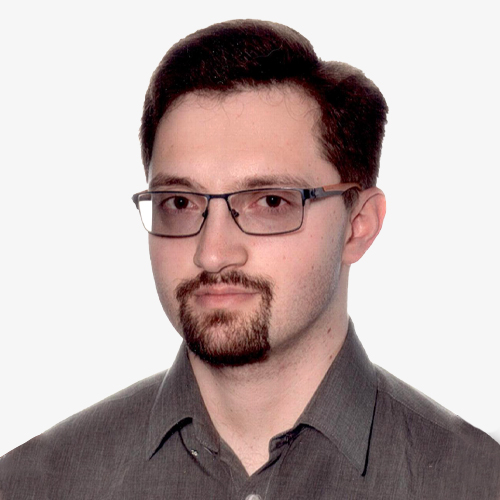
Wiktor Beker, PhD
Senior AI Specialist
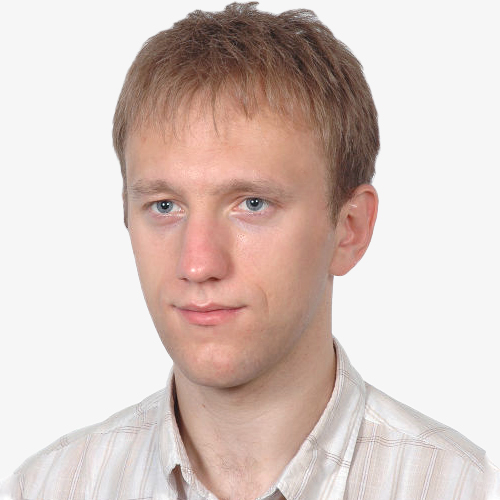
Rafał Roszak, PhD
Senior Software-Development Specialist
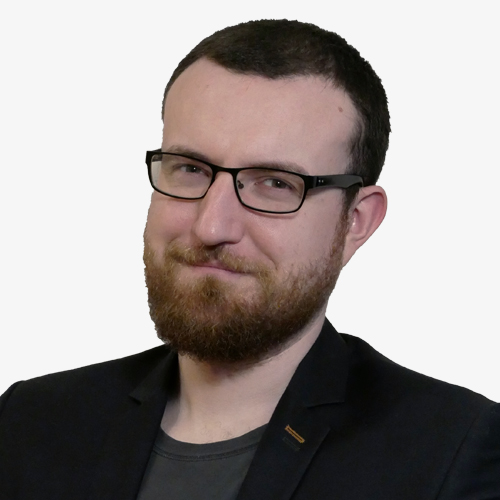
Tomasz Klucznik, PhD
Senior Chemist, computational reaction design
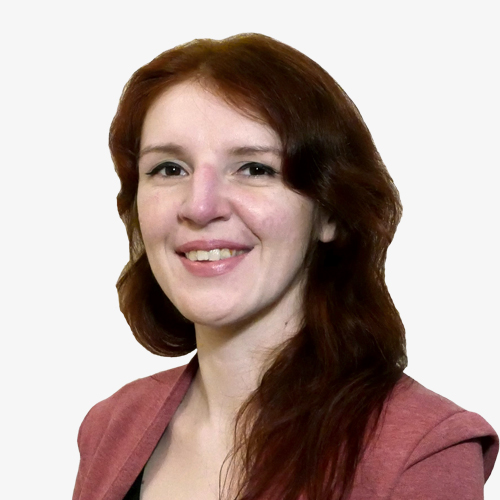
Barbara Mikulak-Klucznik, PhD
Senior Chemist, computational reaction design
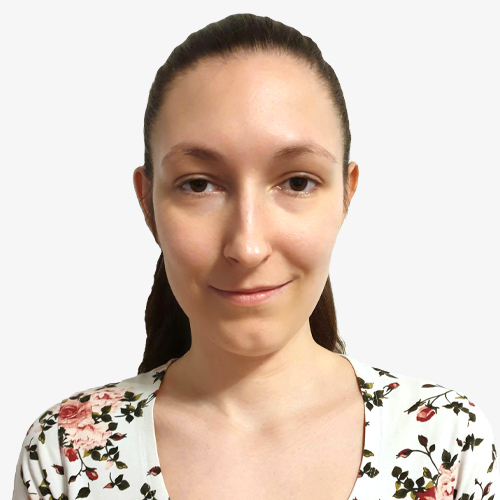
Martyna Moskal
Senior Software Engineer
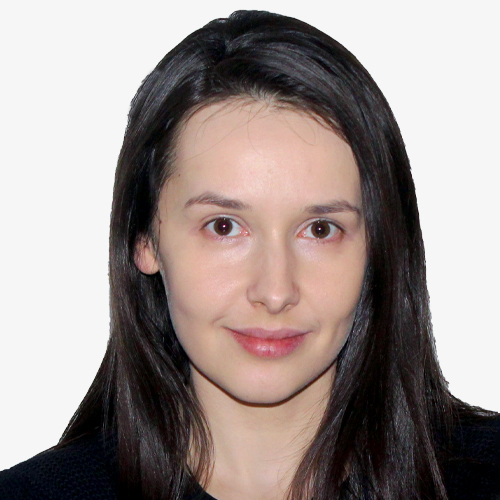
Sara Szymkuć, PhD
Co-Founder and President
News
August 2024
Let’s Meet at the 2024 ACS Fall Meeting!
We’re excited to announce that Dr. Szymkuć will be giving a talk at the 2024 ACS Fall Meeting in Denver. It’s a great opportunity to connect and discuss the latest advancements in computer-assisted chemical synthesis and discovery. For more details, click here.
August 2024
We are pleased to announce a strategic distribution agreement with Bruker SciY™! The innovative SciY™ Platform offers a wide range of scientific and automation software solutions for the digital transformation of the life science and biopharma industries.
Read the full press release here.
Stay tuned for more updates on this exciting partnership!
April 2024
Our latest Research covered by Forbes Magazine!
The Allchemy team’s publication about AI-supported fentanyl production (helping governmental agencies fight the illicit use of the drug) was discussed in the Forbes Magazine article. You can read the full story here.
We are thrilled that our research is reaching the broader community through such a prestigious magazine!
April 2024
Check out our newest JACS paper!
In the article we discuss challenges of computer-assisted planning of complex multistep chemical syntheses and the role of AI algorithms involved in the process. As it turns out, not only reaction example data but also strong expert knowledge is necessary to create reliable retrosynthetic software capturing all nuances of organic syntheses.
The full paper is available here.
February 2024
Our latest scientific article on AI-supported fentanyl production is now published in Chem journal. Through this research we are helping governmental drug agencies to fight the fentanyl crisis by predicting possible illicit syntheses of fentanyl and its analogs.
The full paper is available here.
November 2023
Our new Nature is out!
We are proud to announce that the latest research from Allchemy was published in Nature magazine!
The innovative algorithms within our newest HopCat tool predict mechanisms and outcomes of complex cationic rearrangements by integrating mechanistic knowledge, physical-organic rules, and quantum mechanical calculations. The tool, which has been extensively validated through experiments, holds the potential to revolutionize the prediction and analysis of intricate chemical reactions.
For more details, you can access the full paper here.
August 2023
We are pleased to share that Dr. Szymkuć will be a keynote speaker at the IUPAC|CHAINS 2023 conference! This significant event, held in The Hague, Netherlands, will bring together leading researchers and professionals in the field of chemistry.
Dr. Szymkuć’s speech will focus on advancements in the computer-designed revalorization and controlled degradation of chemical wastes, contributing valuable insights to this important area of research.
November 2022
We are proud to share the news that our latest research from Allchemy, in collaboration with Burke’s lab, has been published in Science magazine!
Within the research, being a part of the DARPA AMD program, we demonstrated a novel closed-loop optimization workflow to discover general conditions for challenging Suzuki-Miyaura cross-coupling reaction. This new approach combines data-guided matrix down-selection, uncertainty-minimizing machine learning, and robotic experimentation to double the average yield of reactions compared to benchmark conditions!
Read the details here.
October 2019
Our new Nature paper is out!
We are proud to announce the publication of our latest research, performed in collaboration with ODP Pharmaceuticals, in Nature magazine. This work demonstrates a novel approach to address chemical waste by transforming them into valuable APIs. Using the Allchemy platform, we mapped out extensive synthetic pathways from approximately 200 industrial waste molecules to over 300 important drugs and agrochemicals. ODP Pharmaceuticals synthesized some of the software’s predictions on a kilogram scale, validating the practical application of the computational findings. The research showcases the potential of computational tools in achieving sustainable and efficient chemical recycling contributing for a greener future in the chemical industry.
For more details, you can access the full paper here.
March 2022
We are excited to share the news about our latest paper being published in the Journal of the American Chemical Society (JACS), in collaboration with Burke’s Lab. Our research shows the limitations of AI methods when learning from literature data, specifically in predicting optimal reaction conditions for heterocyclic Suzuki–Miyaura coupling reactions.
In the study, we demonstrate that even with extensive, carefully curated datasets, machine learning models often fail to outperform simple predictions based on the popularity of reported conditions. These findings highlight the importance of generating standardized and reliable datasets to improve the accuracy and applicability of AI in synthetic chemistry.
Interested in learning more about the intricacies of our research and its implications? Check out the full details here.
December 2020
Our President, Dr. Szymkuc interviewed by Nature and National Geographic!
October 2020
Allchemy commences Phase II of DARPA’s Accelerated Molecular Discovery, AMD, program. We are working as part of the MADNESS team – why “MADNESS”? Well, check out our partners in crime: Aspuru-Guzik, Cronin, Hein and Burke’s labs! Our algorithms and their robots are hell of a madness mix!
August 2020
We are proud to become an Affiliate of the Molecule Maker Lab Institute, one of only five AI Centers funded by the NSF. We will be working with colleagues at Urbana-Champaign to develop new robotized chemistries and also new catalysts. Fun science ahead!
August 2020
Our AI is spot on when it comes to distinguishing drug-like from non-drug-like molecules! Read our newest paper in Nature Machine Intelligence
August 2019
Work is underway! We are excited to announce that Allchemy has commenced effort on two DARPA-sponsored projects, one with Lawrence Livermore National Laboratory and one within the Accelerated Molecular Discovery program.
Publications
Science
“Delocalized, Asynchronous, Closed-Loop Discovery of Organic Laser Emitters.”
Strieth-Kalthoff, F.; Hao, H.; Rathore, V.; Derasp, J.; Gaudin, T.; Angello, N. H.; Seifrid, M.; Trushina, E.; Guy, M.; Liu, J.; Tang, X.; Mamada, M.; Wang, W.; Tsagaantsooj, T.; Lavigne, C.; Pollice, R.; Wu, T. C.; Hotta, K.; Bodo, L.; Li, S.; Haddadnia, M.; Wołos, A.; Roszak, R.; […] Grzybowski, B. A.; Cronin, L.; Hein, J. E.; Burke, M. D.; Aspuru-Guzik, A.
Chem
“Catalyst: Curtailing the Scalable Supply of Fentanyl by Using Chemical AI”
Mikulak-Klucznik, B.; Klucznik, T.; Beker, W.; Moskal, M.; Grzybowski, B. A.
Nature Synthesis
“Computational Synthesis Design for Controlled Degradation and Revalorization”
Żądło-Dobrowolska, A.; Molga, K.; Kolodiazhna, O. O.; Szymkuć, S.; Moskal, M.; Roszak, R.; Grzybowski, B. A.
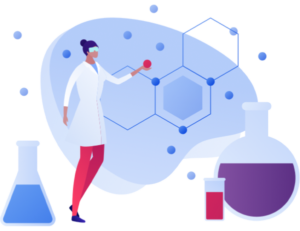
Journal of American Chemical Society
“Artificial Intelligence for Retrosynthetic Planning Needs Both Data and Expert Knowledge”
Strieth-Kalthoff, F.; Szymkuć, S.; Molga, K.; Aspuru-Guzik, A.; Glorius, F.; Grzybowski, B. A.
Chem
“Emergence of Metabolic-like Cycles in Blockchain-Orchestrated Reaction Networks”
Roszak, R.; Wołos, A.; Benke, M.; Gleń, Ł.; Konka, J.; Jensen, P.; Burgchardt, P.; Żądło-Dobrowolska, A.; Janiuk, P.; Szymkuć, S.; Grzybowski, B. A.
Nature
“Computational Prediction of Complex Cationic Rearrangement Outcomes”
Klucznik, T.; Syntrivanis, L.-D.; Baś, S.; Mikulak-Klucznik, B.; Moskal, M.; Szymkuć, S.; Mlynarski, J.; Gadina, L.; Beker, W.; Burke, M. D.; Tiefenbacher, K.; Grzybowski, B. A.
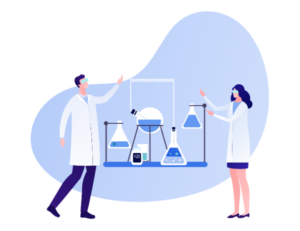
Science
“Closed-Loop Optimization of General Reaction Conditions for Heteroaryl Suzuki-Miyaura Coupling”
Angello, N. H.; Rathore, V.; Beker, W.; Wołos, A.; Jira, E. R.; Roszak, R.; Wu, T. C.; Schroeder, C. M.; Aspuru-Guzik, A.; Grzybowski, B. A.; Burke, M. D.
Nature
“Computer-Designed Repurposing of Chemical Wastes into Drugs”
Wołos, A.; Koszelewski, D.; Roszak, R.; Szymkuć, S.; Moskal, M.; Ostaszewski, R.; Herrera, B. T.; Maier, J. M.; Brezicki, G.; Samuel, J.; Lummiss, J. A. M.; McQuade, D. T.; Rogers, L.; Grzybowski, B. A.
Nature
Beker, W.; Roszak, R.; Wołos, A.; Angelo, N. H.; Rathore, V.; Burke, M. D; Grzybowski, B. A.
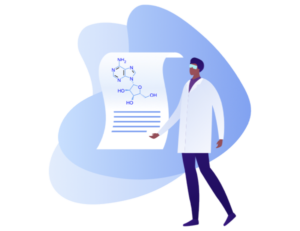
Science
“Synthetic connectivity, emergence, and self-regeneration in the network of prebiotic chemistry”
A. Wołos, R. Roszak, A. Żądło-Dobrowolska, W. Beker, B. Mikulak-Klucznik, G. Spólnik, M. Dygas, S. Szymkuć, B. A. Grzybowski
Nature Machine Intelligence
“Minimal-uncertainty prediction of general drug-likeness based on Bayesian neural networks”
W. Beker, A. Wołos, S. Szymkuć, B. A. Grzybowski
Journal of American Chemical Society
“Rapid and accurate prediction of pKa values of C–H acids using Graph Convolutional Neural Networks”
R. Roszak, W. Beker, K. Molga, B. A. Grzybowski
Science
“Synthetic connectivity, emergence, and self-regeneration in the network of prebiotic chemistry”
A. Wołos, R. Roszak, A. Żądło-Dobrowolska, W. Beker, B. Mikulak-Klucznik, G. Spólnik, M. Dygas, S. Szymkuć, B. A. Grzybowski
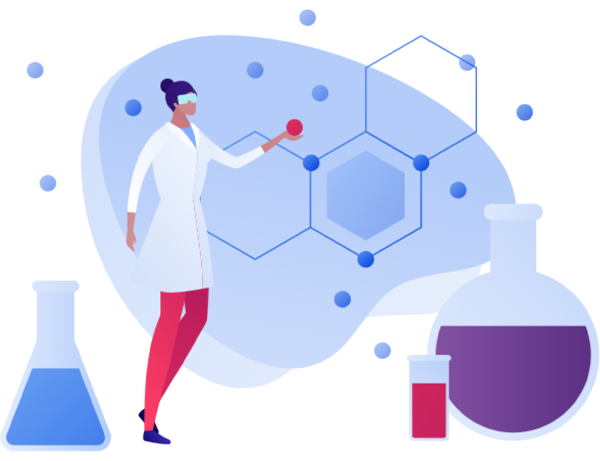
Nature Machine Intelligence
“Minimal-uncertainty prediction of general drug-likeness based on Bayesian neural networks”
W. Beker, A. Wołos, S. Szymkuć, B. A. Grzybowski
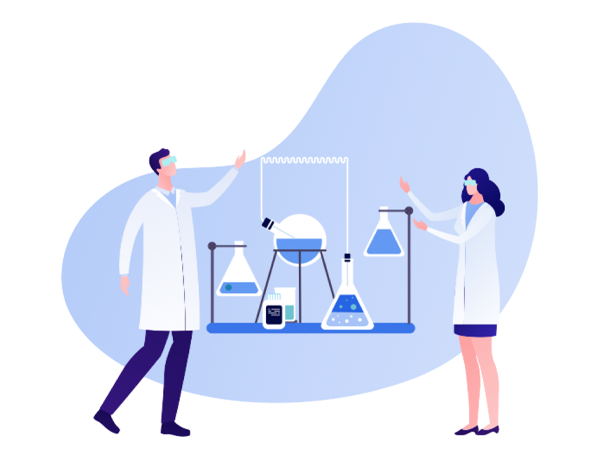
Journal of American Chemical Society
“Rapid and accurate prediction of pKa values of C–H acids using Graph Convolutional Neural Networks”
R. Roszak, W. Beker, K. Molga, B. A. Grzybowski
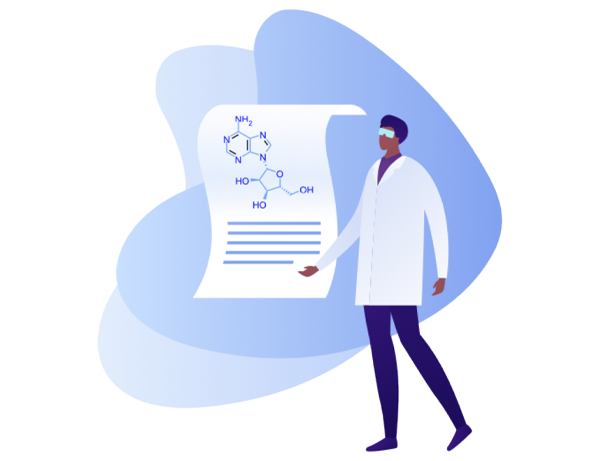